Navigating barriers and paving the way for GenAI in insurance
6-minute read
Published on: 2 January 2025
Gen AI is revolutionizing the insurance industry. However, with great power comes great responsibility. As AI continues to evolve, forward-thinking firms have an opportunity to enhance their operations across several key areas, but this potential also brings concerns that need to be addressed by insurers.
The rapid growth of AI and machine learning in managing claims, underwriting, and other processes introduces new challenges related to data security, ethics, and consumer protection. These concerns are increasingly influencing buyer and regulator attitudes, making it imperative for insurers to navigate these barriers effectively.
In our recent webinar, The AI Edge in Insurance, we examined AI-related challenges and opportunities ahead for the industry.
What are the challenges of AI adoption?
One of the most striking aspects of AI integration in recent years is the predominance of public-facing implementations. Consumer-grade tools like ChatGPT have given everyday users firsthand experience with the power of Generative AI (GenAI) products. Much of this progress is built on Large Language Models (LLMs), which despite their sophistication, remain difficult to understand for those on the outside looking in.
To gain insight into industry sentiments, we polled our webinar audience about their biggest concerns regarding Gen AI implementation in insurance.
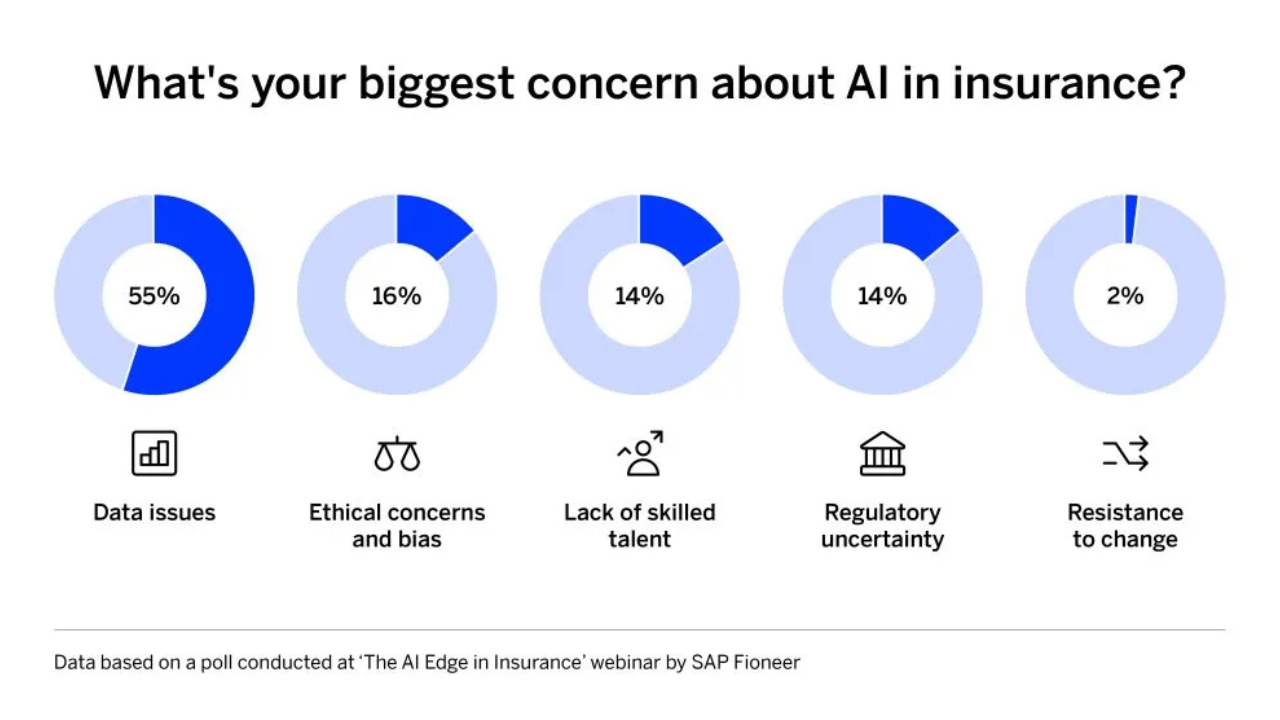
The results were telling and below, we examine each aspect in greater detail.
1. Data quality
The insurance industry thrives on vast amounts of data, from customer preferences to risk models used in underwriting policies. However, this abundance introduces challenges in data management, including volume, quality concerns, siloed systems, and integration difficulties. With data quality and security forming the bedrock of effective AI systems, Madhu Malhotra, CTO at Zuno General Insurance, emphasized, “If the data is accurate, has integrity, and incorporates all the organization’s key metrics, then it’s the right base to start with.”
AI models rely on high-quality, structured data. Issues in integrating legacy systems with modern AI technology can lead to data inconsistencies and accessibility issues, ultimately limiting AI performance.
The other side of data accessibility is security. Insurers must understand what happens when information enters an LLM, and ensure it doesn’t inadvertently expose sensitive data. Clear boundaries must be established to protect proprietary data from being used to train external models or being exposed to competitors.
2. Ethical concerns and algorithmic bias
Beyond data concerns, ethical issues like algorithmic bias present significant challenges.
The ‘black box’ nature of AI systems – the inability to fully understand how they arrive at decisions – can increase ethical risk in critical judgments.
As Jon Holvoet, CTO of Credendo, warned: “Explainability of the AI models is becoming a big challenge, especially as deep learning models evolve.” The complexity of these models raises concerns about understanding their decision-making processes – especially if biases or discrimination are embedded in the training data.
A recent Ethical AI in Insurance Consortium Survey found that 69% of respondents were dissatisfied with their current processes to manage and address biases in AI models.
There’s growing pressure on firms to meet stringent safety requirements imposed by regulators concerning customer data privacy and fairness, particularly in areas like underwriting and claims approval.
3. Talent
From April 2022 to April 2023, online job listings for AI-focused roles surged 75-fold. However, as Jon noted: “AI is not a magical bullet in underwriting… you still need the human in the loop for final validation.”
Regardless of AI’s power, responsibility and accountability for outcomes will rest with the business, both ethically and legally.
“Lack of skilled talent is definitely a challenge. AI is new, and it’s going to take some learning. We’re all on the journey, learning about AI and the required skills, and that’s something we need to overcome,” said Jon. Insurers must broaden their search for talent and compete with other industries vying for the same skill sets.
Successful AI deployment requires synergy between human expertise and AI capabilities. Human oversight is crucial to managing ethical concerns, ensuring accuracy, and adapting AI recommendations based on nuanced industry knowledge.
4. The regulatory landscape
The Asia-Pacific (APAC) region has been a first mover in AI regulation, though approaches vary significantly between countries.
- Mainland China leads with targeted regulations, including measures for deepfakes, recommendation algorithms, and generative AI services.
- Japan and Australia are developing frameworks based on ethical principles and risk assessment while considering AI-specific legislation.
- Hong Kong and Singapore rely on existing laws supplemented with sector-specific guidance, focusing on transparency, fairness, and data privacy.
Likewise, the Financial Conduct Authority (FCA) and the Prudential Regulation Authority (PRA) are leveraging existing consumer protection laws to regulate AI usage in the UK insurance industry The European Union’s AI Act, set to come into force in 2026, will further impact regulatory requirements.
New rules will require insurers to balance their enthusiasm for new tools with a responsible approach to how their models perform, including appropriate testing before launch.
A responsible approach to implementation
To maximize the benefits of AI-driven systems, insurers need to invest in the right infrastructure and strategic considerations to integrate these tools alongside existing processes.
Pilot Programs and Iterative Testing
Before mass implementation, small-scale pilots can help identify ethical or regulatory issues. Working in secure, contained testing environments allows insurers to refine AI applications, test integrations, outputs, and workflows, ensuring compliance and alignment with project goals. Successful AI implementation must be anchored in a strategic vision and a well-defined roadmap. This includes investing in data, technology, and talent aligned with business objectives while striving for reproducible results. McKinsey highlights focusing on reusing scalable components across different AI applications to accelerate development and integration, creating a system of common elements that can be proven and approved across different use cases.
Investment in skilled talent
Ensuring security, efficiency, and ethical integrity requires the right talent to build and monitor these systems. Investing in AI experts and data scientists who can collaborate with industry specialists is crucial.
Although AI expertise is in high demand across industries, insurance offers valuable opportunities in terms of scale, data breadth, and human impact, which could be attractive for AI professionals.
Collaborative approaches to growth
In the race to make the most of AI, insurers must balance development speed with responsible, efficient methods that mitigate risks such as data breaches, rogue outputs, and system failures.
Rapidly updating infrastructure and expertise can strain internal resources, given the specialized tools and expertise required. Collaborating with external technology partners such as SAP Fioneer can accelerate time to value, and it starts with investing in the right infrastructure to enable effective synergy between AI systems and human expertise.
Balancing risk and opportunity in AI
The race for AI implementation in insurance is well underway, with use cases emerging across the industry. However, success isn’t just about being first, it’s about moving forward thoughtfully. Rushing without addressing inherent risks can expose insurers to regulatory action, consumer backlash, and losses from unproductive development projects.
Recognizing the power of AI can cut both ways, insurers must prepare their strategies accordingly. By addressing data quality and security, ethical considerations, talent acquisition, and regulatory compliance, insurers can navigate the barriers and pave the way for GenAI—building on the opportunities outlined in our previous discussion.
Webinar: The AI Edge in Insurance
Learn how AI is revolutionizing every aspect of insurance operations, practical challenges of developing and deploying AI within insurance operations, and transferrable learnings from other industries.
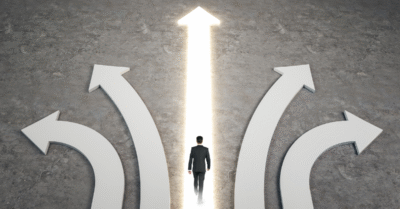
Related posts

Mapping the true potential of GenAI: Where it counts in insurance

Investing in AI boundaries key to incorporating GenAI across insurance value chain

Getting personal: How AI can revolutionize personalization in insurance
Most read posts

The modernization dividend: Leveraging core insurance system upgrades for growth

Virtual account management: the quick win for a stronger cash management proposition

The new role of CFOs in banks: How AI is shaping a new era of finance
More posts
Get up to speed with the latest insights and find the information you need to help you succeed.