Mapping the true potential of GenAI: Where it counts in insurance
6-minute read
Published on: 19 November 2024
With the advent of large language models (LLM), GenAI systems have accelerated innovation in the insurance industry. This impacts areas from risk assessment and pricing, product and policy management to claims processing and customer service.
A recent survey found that 77% of insurers are in some stage of adopting AI in their value chain, particularly Generative AI. And 59% of insurers are actively exploring or implementing solutions.
While the flexibility and power of GenAI hold immense potential, realizing its precise impact requires a strategic approach. It requires balancing technological capability with regulatory, ethical, and business considerations.
As Chirag Shah, SAP Fioneer’s Managing Director of Insurance, highlighted in our recent webinar, The AI Edge in Insurance, “AI will have an impact across the value chain in the insurance space… but AI will not have the same impact across all areas.”
Joining Chirag were Jon Holvoet, Chief Technology Officer at Credendo, and Madhu Malhotra, Chief Technology Officer at Zuno General Insurance, to discuss real-world use cases for AI in insurance. Here, we explore their insights and recommendations on steps to bring an AI-driven future into focus.
Where are the current opportunities for GenAI in insurance?
There have been rapid changes in consumer preferences, business models, and technology standards. Therefore, insurers face increasing pressure to innovate their products, enhance customer experiences, and improve operational efficiency. AI has been embraced swiftly for its ability to streamline transformation. This has enabled more responsive and agile insurance services without driving up costs. Our webinar highlighted several use cases that are already in practice in the industry.
a. Implementing customer-focused risk assessment and pricing
Risk assessment and pricing involve analyzing numerous data points on an individual customer basis. However, the effort and cost of this analysis can limit insurers’ ability to tailor policies effectively. PwC highlights that carriers could see actual loss ratios increase by 1–2% every quarter without more efficient pricing metrics.
In order to address these challenges, AI-driven data analysis enhances accuracy in risk assessment, enabling insurers to customize pricing more effectively. Likewise machine learning models can analyze customer behavior and external factors in real time, optimizing pricing strategies without extensive manual review.
Illustrating this point, Madhu Malhotra shared that Zuno Insurance is already using AI to analyze loss ratios and trigger mechanisms for potential risks. This has improved its ability to assess and mitigate risks accurately.
b. Personalizing for product and policy management
Personalized services have become increasingly common in both consumer and B2B sectors. Our recent buying report found that 94% of 18-34 year-olds would consider switching to an insurance provider that uses advanced data analytics for personalized policies.
AI opens the door to offering personalized insurance solutions at the point of policy creation. This allows insurers to meet the needs of diverse customer segments more precisely. This has a tangible commercial impact, as Madhu highlighted, “AI-driven hyper-personalization is becoming a key differentiator for us, helping us better engage customers and offer products that are suited to their specific needs.”
For example, by implementing AI in usage-based car insurance products, which segment customers based on their driving behavior, Zuno can create more tailored profiles and specific offers for customers with high scores, aligning better with their personal risk profiles.
c. Streamlining claims processing and fraud detection
In a traditional workflow, growth in customer volumes increases the risk for insurance companies. A higher volume of claims puts more pressure on internal teams to process claims quickly. This raises fraud risk due to overstretched resources, which is significant. Financial institutions in APAC incur an estimated loss of $4.59 for every Singapore dollar lost to fraud.
Automation through AI can both decrease risk and improve back-office efficiency by accelerating claims handling, reducing processing times, and improving accuracy. AI also supports fraud detection, reducing operational risks and ensuring a smoother customer experience.
These tools are already in place at Zuno. From the First Notification of Loss (FNOL), where half of all claims are registered via AI, to fraud detection, AI analyzes patterns and anomalies in both historical and customer data to highlight suspicious patterns and augment human review.
Meanwhile, at Credendo, AI supports review teams with better, faster data. “We’re using machine learning in the analysis of unstructured financial data in the underwriting process, and as an aid in AI document translations for underwriters,” said Jon Holvoet.
Subsequently, this enables underwriters to increase their efficiency on a per-client basis while working with more reliable data points in the document-heavy underwriting process.
d. Simplifying customer service
The rise of embedded insurance – offering insurance at the point of sale – has increased pressure on insurers to compete on customer experience. Investing in service quality is essential to retain customers in the long term.
Customer service is a common pain point, with 63% of customers reporting frustration with their insurance providers. AI-driven chatbots and virtual assistants can improve service access for customers. This provides around-the-clock support and enhancing customer interaction while reducing the workload on human agents.
Zuno has successfully implemented an automated first stage of claim submission: “At Zuno, 50% of all claims are registered through AI voice bots, making the process quicker and more efficient,” Madhu noted. As a result, this allows customers to submit claims without having to wait for an agent. It then allows internal teams to focus more on claim review rather than document management.
Building a roadmap for future use cases
As AI continues to evolve rapidly, more opportunities will emerge with the development of complementary solutions within the insurance industry. In our work with leading insurers, SAP Fioneer is exploring the next stage of foundational technology. Our goal is to drive new use cases in the industry, including:
- Fraud detection: enhancing broader back-office operations, including payments and commissions management by detecting unusual commission payments to agents and flagging potential issues requiring human attention.
- Product development: Using AI to help insurers create complex product structures, improving speed and reducing manual effort in designing insurance products tailored to specific customer needs.
- AI development: Leveraging AI to improve the creation and accuracy of AI products themselves, where SAP Fioneer aims to boost development productivity by 60-70%.
At this early stage of AI implementation, progress is a conversation between technological capabilities, customer expectations, and the ambition of insurers themselves to push for better, more competitive processes. Closer collaboration and feedback among these spheres will enable greater achievements. Insurers must listen closely and act proactively, or they may find that the future they’re looking for has already arrived.
Webinar: The AI edge in insurance
Learn how AI is revolutionizing every aspect of insurance operations, practical challenges of developing and deploying AI within insurance operations, and transferrable learnings from other industries. Watch the webinar on-demand.
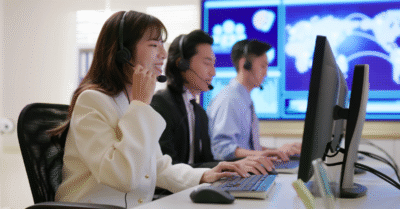
Related posts

Unlocking scalable AI in insurance from the core

Navigating barriers and paving the way for GenAI in insurance

Investing in AI boundaries key to incorporating GenAI across insurance value chain
Most read posts

The modernization dividend: Leveraging core insurance system upgrades for growth

Navigating barriers and paving the way for GenAI in insurance

Virtual account management: the quick win for a stronger cash management proposition
More posts
Get up to speed with the latest insights and find the information you need to help you succeed.